Research Projects
Open Projects
Social Impact of Long-Term Cooperation With Robots July 1, 2013 - Present
Director: David Feil-Seifer
SAR envisions robots acting in very human-like roles, such as coach, therapist, or partner. This is a sharp contrast from manufacturing or office assistant roles that are more well-understood. In some cases, we can use human-human interaction as a guide for HRI; in others, human-computer interaction can be a suitable guide. However, there may be cases where neither human-human nor human-computer interaction provide a sufficient framework to envision how people may react to a robot over a long term. Empirical research is required in order to examine these relationships in more detail.
Preliminary work suggests that a person sometimes views a robot as very machine-like, but others suggest that a robot can take on a social role. Long-term interaction between humans and robots might also expose previously unforeseen challenges for creating and sustaining relationships. Experiment design experts suggest that participants in lab studies might subconsciously change their reactions to the robot given certain assumptions that arise from being in a research setting. This could have ramifications for in-lab research related to lt-HRI, requiring out-of-lab validation as well. This objective will study these and other social factors related to lt-HRI to observe how they may impact acceptance and cooperation.
Empirical Study of Socially-Appropriate Interaction July 1, 2013 - Present
Director: David Feil-Seifer
The goal of this project is to investigate how human-robot interaction is best structured for everyday interaction. Possible scenarios include work, home, and other care settings where socially assistive robotics may be used as educational aids, provide aid for persons with disabilities, and act as therapeutic aids for children with developmental disorders. In order for robots to be integrated smoothly into our daily lives, a much clearer understanding of fundamental human-robot interaction principles is required. Human-human interaction demonstrates how collaboration between humans and robots may occur. This project will study how socially appropriate interaction and, importantly socially-inappropriate interaction can affect human-robot collaboration.
In order to explore the nature of collaborative HRI for long-term interaction, REU participants will work with project personnel to develop controlled experiments which isolate individual aspects of collaborative HRI such as conformity, honesty, mistreatment, and deference. Students will examine these factors in a single-session experiment that they design. The results from these experiments will be used to focus multi-session follow-up studies that will be conducted in-between summers. The results of this research project will be a compendium of data demonstrating how humans and robots interact in situations of daily life, such as manufacturing, education, and daily living.
Socially-Aware Navigation Sept. 1, 2017 - Present
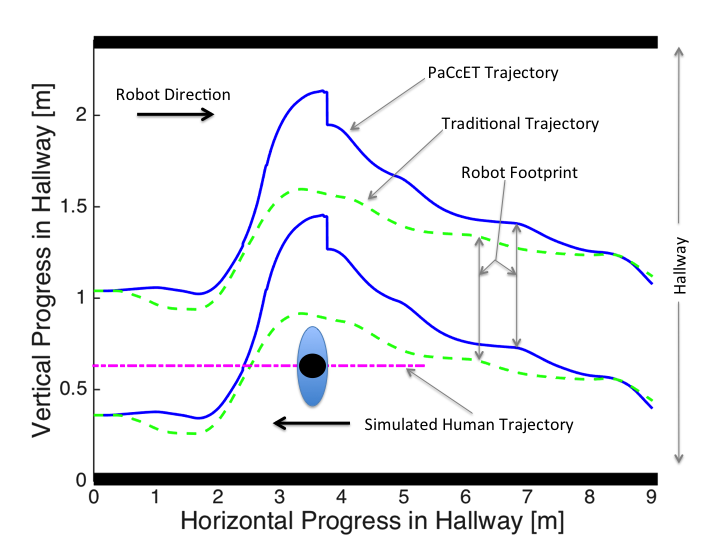
Director: David Feil-Seifer
As robots become more integrated into people's daily lives, interpersonal navigation becomes a larger concern. In the near future, Socially Assistive Robots (SAR) will be working closely with people in public environments. To support this, making a robot safely navigate in the real-world environment has become for active study. However, for robots to effectively interact with people, they will need to exhibit socially-appropriate behavior as well. The real-world environment is full of unpredictable events; the potential social cost of a robot not following social norms becomes high. Robots that violate these norms risk being isolated and falling out of use, or even being mistreated by their human interaction partners.
The focus of this work is to develop a people-aware navigation planner to handle a larger range of person-oriented navigation behaviors utilizing a multi-modal distribution model of human-human interaction. Interpersonal distance models based on features which could be detected real-time using on-board robot sensors can be used to discriminate between a set of human actions and provide a social appropriateness score for a potential navigation trajectory. These models are used to weigh the trajectories and select the most appropriate action for the given situation. This robot navigation mimics the adherence to social norms while simultaneously adhering to a stated navigation goal as well. The goal of this system is to sense interpersonal distance and choose a trajectory that jointly optimizes for both social-appropriateness and goal-orientedness.
Distributed Control Architecture for Collaborative Multi-Robot Task Allocation March 1, 2016 - Present
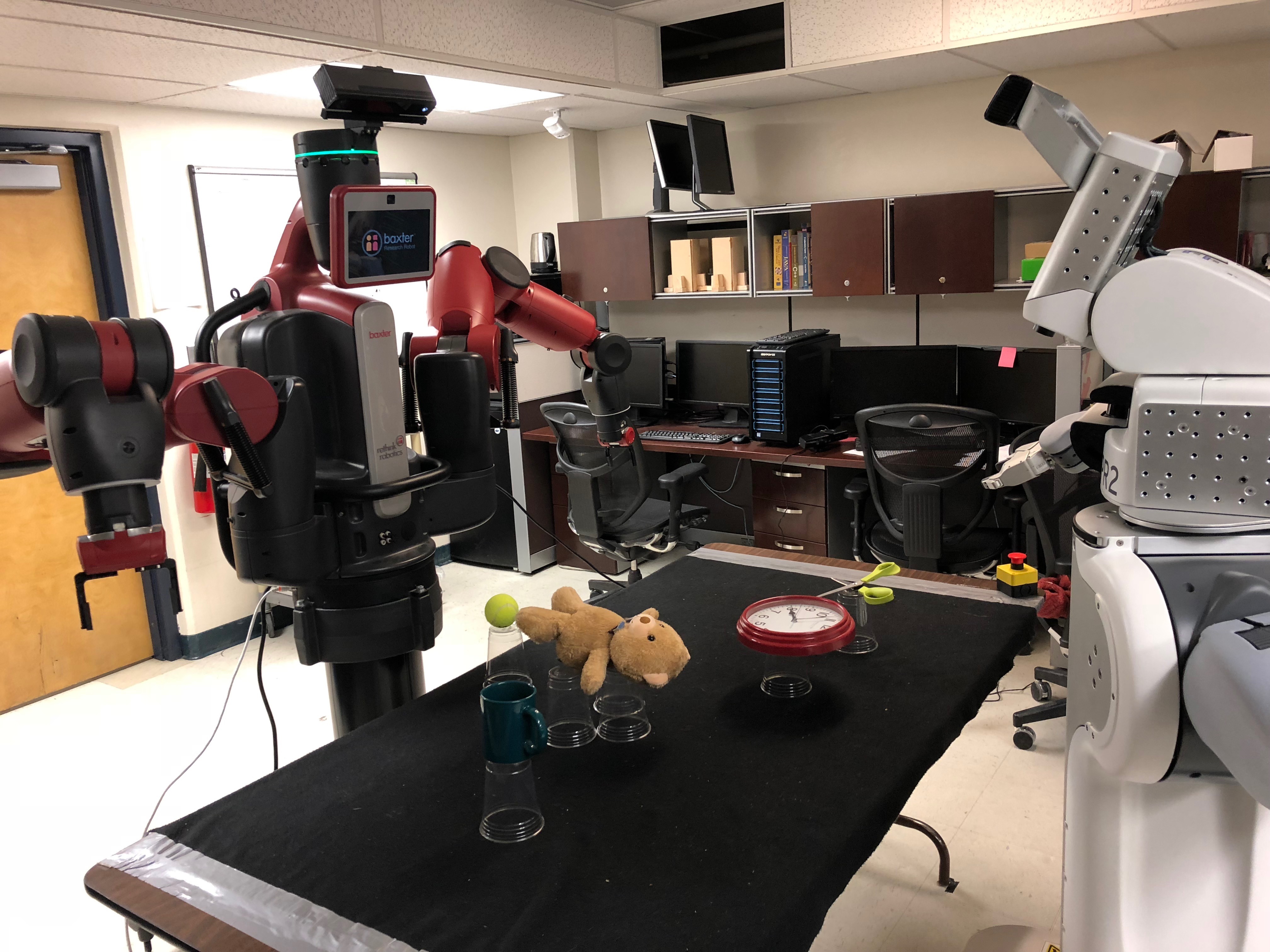
Director: Monica Nicolescu
Real-world tasks are not only a series of sequential steps, but typically exhibit a combination of multiple types of constraints. These tasks pose significant challenges, as enumerating all the possible ways in which the task can be performed can lead to large representations and it is difficult to keep track of the task constraints during execution. Previously we developed an architecture that provides a compact encoding of such tasks and validated it in a single robot domain. We recently extended this architecture to address the problem of representing and executing tasks in a collaborative multi-robot setting. Firstly, the architecture allows for on-line, dynamic allocation of robots to various steps of the task. Secondly, our architecture ensures that the collaborative robot system will obey all of the task constraints. Thirdly, the proposed architecture allows for opportunistic and flexible task execution given different environmental conditions. We demonstrated the performance of our architecture on a team of two humanoid robots (a Baxter robot and a PR2 robot) performing hierarchical tasks. Further extensions of this architecture are currently being explored.
Due to the adaptability of our multi-robot control architecture, this work has become the foundation for several ongoing extensions. The first extension is to modify the architecture to allow for a human to collaborate with the multi-robot team in order to complete the joint task. To allow the human and robots to communicate, this work augments the control architecture with a third robot “brain” which utilizes intent recognition to determine which objects the human is interacting with and updates the state of the task held by the other robots accordingly. Secondly, this architecture is being adapted to allow a human to train a robot to complete a task through natural speech commands. The multi-robot team is then able to complete the newly trained task. The third extension focuses on learning generalized task sequences utilizing the types of hierarchical constraints defined by this control architecture. The last extension to this work is to modify the architecture to allow heterogenous grasp affordances for each item in the task. These affordances allow the robots to incorporate how well they can grasp a specific object based on their gripper and arm mechanics into the task allocation architecture. This allows robots to be assigned tasks which they are better suited for. This work is currently being completed and the aim is to submit this work to DARS 2018. Each of these extensions emphasize the generalizability of this control architecture and together illustrate the vast applications in which further extensions of this architecture can be adapted to.
Methods For Facilitating Long-Term Acceptance of a Robot July 1, 2013 - Present
Director: David Feil-Seifer
Scenarios that call for lt-HRI, such as home health care and cooperative work environments, have interesting social dynamics, even before robots are introduced. The success of human-only teams can be limited by the trust and willingness to collaborate within the team, consideration is worthy of factors that may affect adoption of robots in long-term settings. A coworker who is unwilling to collaborate and trust the abilities of their fellow teammate may refuse to include that teammate in many job related tasks due to territorial behaviors. Exclusion of a robotic team member might eventually lead to a lack of productivity in lt-HRI. Behavior toward a robot companion may be governed, in part, by pre-conceived notions about robots. However, prior work has shown that simple team-building exercises can positively affect attitudes toward a robot, even if the robot under-performs at a task. This objective will address these issues by adapting and evaluating facilitation strategies that have been successful in encouraging the adoption of new technology or improving team cohesion. We will study how these facilitation techniques can bolster human-robot cooperation.
Robotics in the Classroom Jan. 1, 2014 - Present
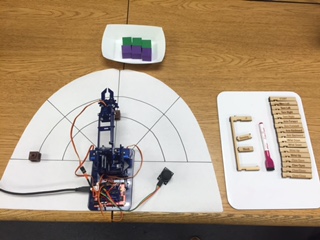
Director: David Feil-Seifer
Robotics represents one of the key areas for economic development and job growth in the US. However, the current training paradigm for robotics instruction relies on primarily graduate study. Some advanced undergraduate courses may be available, but students typically have access to at most one or two of these courses. The result of this configuration is that students need several years beyond an undergraduate degree to gain mastery of robotics in the academic setting. This project aims to: alter current robotics courses to make them accessible for lower-division students; increase both the depth and breadth of the K-12 robotics experience; increase the portability of robotics courses by disseminating course materials online, relying on free and open-source software (FOSS), and promoting the use of simulators and inexpensive hardware. We will team with educators to study the effectiveness of the generated materials at promoting interest in robotics, computing, and technology at the K-5, 6-8, 9-12, and undergraduate grade levels.
Perceptions of Social Intelligence Scale Sept. 1, 2017 - Present
Director: David Feil-Seifer
Social intelligence is the ability to interact effectively with others in order to accomplish your goals (Ford & Tisak, 1983). Social intelligence is critically important for social robots, which are designed to interact and communicate with humans (Dautenhahn, 2007). Social robots might have goals such as building relationships with people, teaching people, learning something from people, helping people accomplish tasks, and completing tasks that directly involve people’s bodies (e.g., lifting people, washing people) or minds (e.g., retrieving phone numbers for people, scheduling appointments for people). In addition, social robots may try to avoid interfering with tasks that are being done by people. For example, they may try to be unobtrusive and not interrupt.
Social intelligence is also important for robots engaged in non-social tasks if they will be around people when they are doing their work. Like social robots, such task-focused robots may be designed to avoid interfering with the work of people around them. This is important not just for the people the robots work with, but also for the robots themselves. For example, if a robotic vacuum bumps into people or scares household pets, the owners may turn it off. In addition, task-focused robots will be better able to accomplish their goals if they can inspire people to assist them when needed. For example, if a delivery robot is trying to take a meal to a certain room in a hospital and its path is blocked by a cart, it may be beneficial if it can inspire nearby humans to move the cart.
While previous research on human-robot interaction (HRI) has referenced and contained aspects of the social intelligence of robots (Bartneck, Kulic, Croft, & Zoghbi, 2009; Ho, MacDorman, 2010; Ho, MacDorman, 2017; Moshkina, 2012; Nomura, Suzuki, Kanda, & Kato, 2006), the concept of robotic social intelligence has not been clearly defined. Measures of similar concepts are brief and incomplete, and often include extraneous variables. Moreover, measures of human social intelligence (e.g., Baron-Cohen, S. Wheelwright ,& Hill, 2001; Silvera, Martinussen, & Dahl, 2001) cannot be adapted for robots, because they assess skills that current and near-future robots do not have and because they omit basic skills that are essential for smooth social interactions. Therefore, we designed a set of 20 scales to measure the perceived social intelligence of robots.
Improving People Detecting Infrastructures for the Purpose of Modeling Human Behavior June 3, 2019 - Present
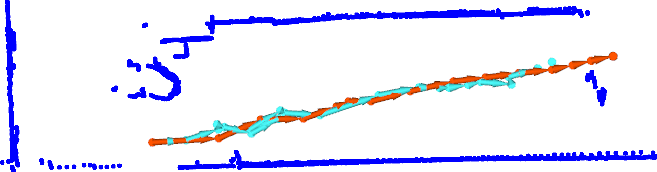
Director: David Feil-Seifer
We need to model human behavior for a better understanding of how robots can behave in crowds. To do that, we can to use lasers to track people’s movements during different social interactions. We have developed a system to enable multiple 2-dimensional, stationary lasers to capture movements of people within a large room during social events. With calibrated lasers, we tracked a moving person’s ankles and predicted his/her path using linear regression. We were also able to overlap all laser fields and convert them into a single point cloud. After comparing the point cloud over five time frames, we correctly detected movement and found ankles in the point cloud with our circle detection algorithm. Using the locations of the tracked ankles, we used linear regression to accurately predict the path of the person. Although the positioning is not perfect, the overall shape of the predicted path resembles the actual path taken by the person. We hope that others will use this system to better understand and model human behavior.
Legibility of Robot Motion in Complex Environments June 1, 2023 - Present
Director: David Feil-Seifer
In human-robot collaboration, legible intent of the robot is critical to success as it enables the human to more effectively work with and around the robot. Environments where humans and robots collaborate are widely varied and in the real world are most often cluttered. However, prior work in legible motion utilizes primarily environments which are uncluttered. Success in these environments does not necessarily guarantee success in more cluttered environments. Furthermore, the prior work has been primarily performed based on results from robot-human studies and the problem has not been studied from the prospective of what people do to express intent to each other. Therefore, this work addresses a gap in current research by studying legible robot arm motion in the context of cluttered environments.
Past Projects
Understanding First Impressions from Face Images June 3, 2019 - March 31, 2020
Director: Emily Hand
This project investigated how first impressions are expressed in faces through the automated recognition of these social traits from face images. First impressions are universally understood to have three dimensions: trustworthiness, attractiveness and competence. All three of these social traits have strong implications for evolution. We form first impressions of sometime within 100ms of meeting them, which is less time than it takes to blink. The first impressions we form are composed of judgements of these three social traits. This project aimed to answer the question: “Can automated methods accurately recognize social traits from images of faces?” Two main challenges are associated with the above research question, and both are related to the data available for the problem. First impressions are not something that can be objectively measured, at this point in time, and so labels for first impressions come from subjective human ratings of faces. Additionally, only one dataset is available for this problem and it has roughly 1,100 images. Training a state-of-the-art machine learning algorithm to accurately and reliably recognize social traits from face images using only 1,100 images each labeled with subjective human ratings is nearly impossible.
Student involvement: Two REU participants investigated a method for combining multiple datasets from different domains with partial labels for the problem of social trait recognition. Using this combined dataset, the students were able to train a model capable of recognizing social traits with improved accuracy over the baseline approach.
FRIDI: First Responder Interface for Disaster Information Aug. 1, 2014 - Dec. 31, 2018
Director: David Feil-Seifer
In recent years robots have been deployed in numerous occasions to support disaster mitigation missions through exploration of areas that are either unreachable or potentially dangerous for human rescuers. The UNR Robotics Research Lab has recently teamed up with a number of academic, industry, and public entities with the goal of developing an operator interface for controlling unmanned autonomous system, including the UAV platforms to enhance the situational awareness, response time, and other operational capabilities of first responders during a disaster remediation mission. The First Responder Interface for Disaster Information (FRIDI) will include a computer-based interface for the ground control station (GCS) as well as the companion interface for portable devices such as tablets and cellular phones. Our user interface (UI) is designed with the goal of addressing the human-robot interaction challenges specific to law enforcement and emergency response operations, such as situational awareness and cognitive overload of the human operators.
Situational Awareness, or otherwise the understanding that the human operator has about the location, activities, surroundings, and the status of the unmanned aerial vehicle (UAV), is a key factor that determines the success in a robot-assisted disaster mitigation operation. With that in mind, our goal is to design an interface that will use pre-loaded terrain data augmented with real-time data from the UAV sensors to provide for a better SA during the disaster mitigation mission. Our UI displays a map of near-live images of the scene as recorded from UAVs, position and orientation of the vehicles in the map, as well as video and other sensor readings that are crucial for the efficiency of the emergency response operation. This UI layout enables human operators to view and task multiple individual robots while also maintaining full situational awareness over the disaster area as a whole.
Disaster Management Simulator Jan. 1, 2016 - Jan. 1, 2018
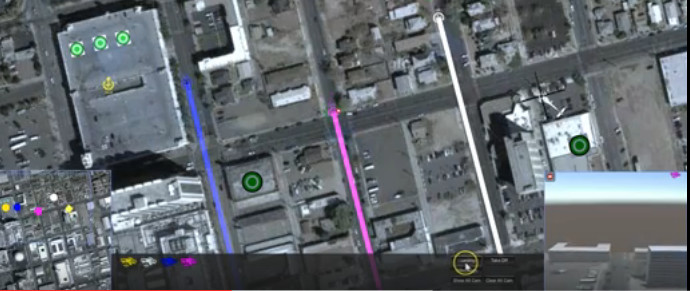
Director: David Feil-Seifer
We are developing a disaster mitigation training simulator for emergency management personnel to develop skills tasking multi-UAV swarms for overwatch or delivery tasks. This simulator shows the City of Reno after a simulated earthquake and allows an operator to fly a simulated UAV swarm through this disaster zone to accomplish tasks to help mitigate the effects of the scenario. We are using this simulator to evaluate user interface design and to help train emergency management personnel in effective UAV operation.
Safety Demand Characteristic June 1, 2016 - Nov. 1, 2017
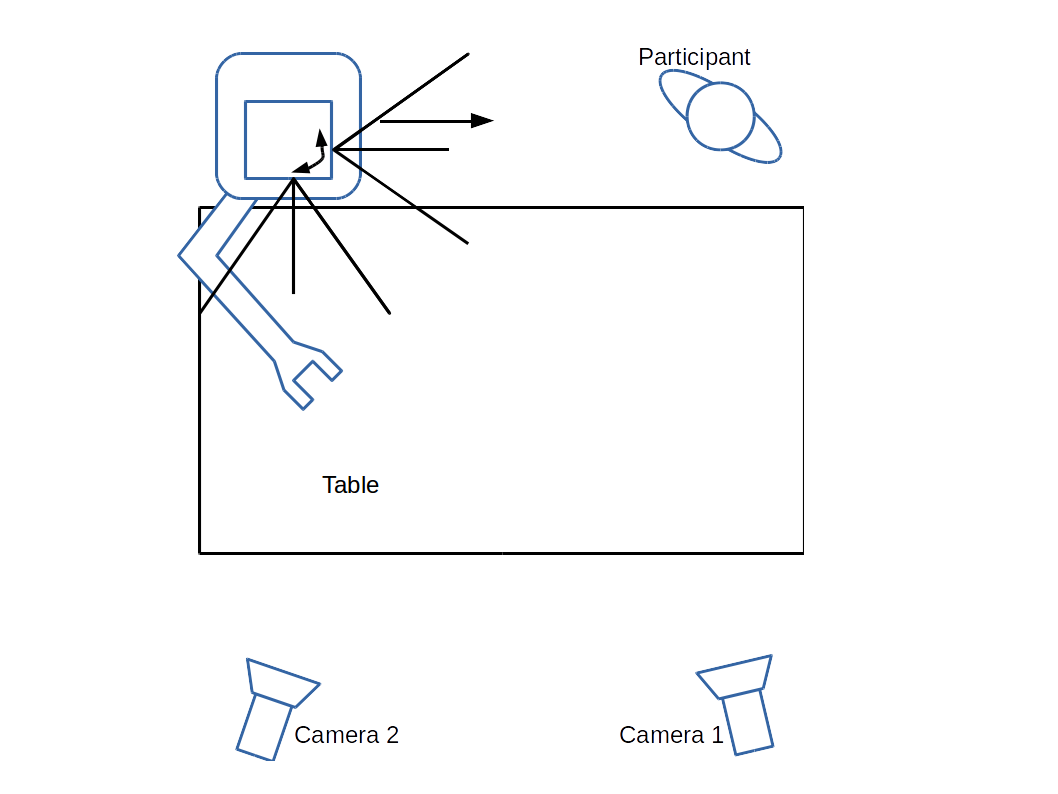
Director: David Feil-Seifer
While it is increasingly common to have robots in real-world environments, many Human-Robot Interaction studies are conducted in laboratory settings. Evidence shows that laboratory settings have the potential to skew participants' feelings of safety. This project probes the consequences of this Safety Demand Characteristic and its impact on the field of Human-Robot Interaction.
Partially Occluded Person Pose Detection July 1, 2014 - Dec. 31, 2016
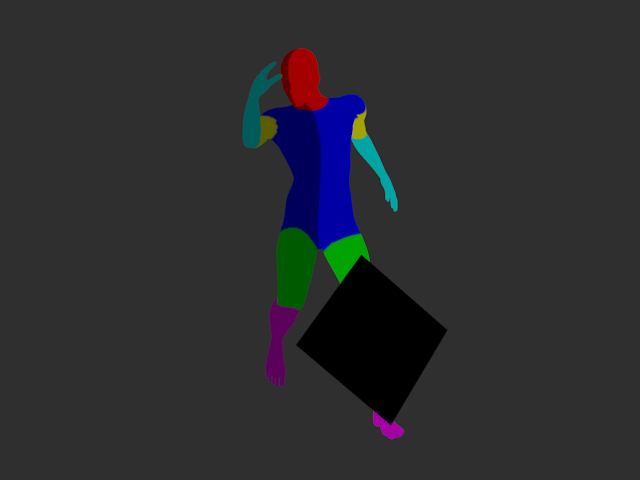
Director: David Feil-Seifer
Detecting humans is a common need in human-robot interaction. A robot working in proximity to people often needs to know where people are around it and may also need to know what the pose, i.e. location of arms, legs, of the person. Attempting to solve this problem with a monocular camera is difficult due to the high variability in the data; for example, differences in clothes and hair can easily confuse the system and so can differences in scene lighting. A common example of a pose detection system is the Microsoft Kinect. The Kinect uses depth images as input for the pose classifier. In a depth image, each pixel has the distance to the nearest obstacle. With the Kinect, users can play games just by moving, since the Kinect can detect their pose; however, the Kinect pose detector is optimized to be used for entertainment. It assumes that the view of the person is completely unobstructed and that the person is standing upright in the frame.
The goal of this project is to create a robust system for person detection. This system will be able to handle detection even when a person is partially occluded by obstacles. It will also make no assumptions about the position of the camera relative to the person. By removing the position assumption, the system will function even when running on a mobile platform, such as a UAV, that may be above or behind the person. The system functions in two stages. In the first stage, the system takes depth images and uses this information to classify every pixel in the image as a certain body part, or non-person, to produce a mask image. This output gives a 2-D view of the scene that shows where each body part is in the image. There are many different techniques for producing the mask image, evaluating the effectiveness of each is one step of the project. In the second stage, the depth data will be combined with the mask image to produce a 3-D representation of the person. That representation will be used to find the position of each body part of interest in 3-D space.